The challenges marketers face overcoming the hyperbole and mysticism that surrounds machine learning and AI
The marketing industry is abuzz with talk of how marketing applications of machine learning and AI will make us more effective and change the way we do our marketing. The problem is that while we’re all keen to embrace innovation and get a competitive edge (which machine learning most definitely provides), for many of us it sounds inaccessible and beyond our typical marketing capability.
However, within the next five years, we believe machine learning will become an everyday skill that marketers will be expected to possess, built into our software and processes as standard. The challenge for marketers today is in overcoming the hyperbole and mysticism that surrounds machine learning and AI, to understand what it might mean to them.
It helps to first look at one of the main issues we marketers face - data. Too much of it and in too many places. I could easily provide you with lots of stats here, such as how IBM says…etc. I find it simpler to examine the data I generate personally.
At this very moment, my phone is in my pocket, creating data about my location and movement. My iWatch is on my wrist taking my heartbeat and tracking how active I am. My laptop and its applications are tracking my behaviours and the things I do (and don’t like to do). Each one of these activities is creating another row of data in someone’s spreadsheet, data which will no doubt be used to sell me (and people like me), more STUFF. Now, times that by millions of people and millions of moments and you begin to get a sense of the amount of data being created all the time.
So what part does machine learning play in this? How can machine learning help us to overcome these issues? Well, it helps to understand the different kinds of decisions people make. We can do that through the Cynefin framework (pronounced Kuh-nevin), developed by IBM in the late 1990s.
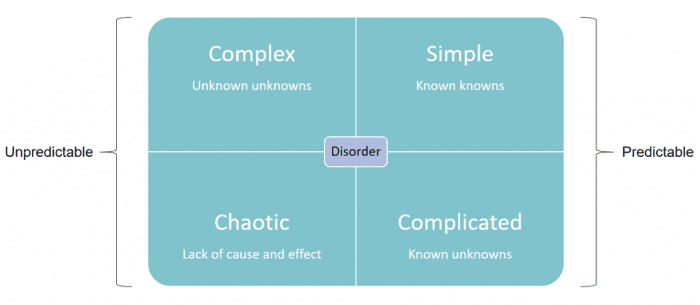
The Cynefin framework tells us that there are five types of decision – simple, complicated, complex, chaotic and disordered. We are going to look at the first four of these.
- Simple decisions represent the known knowns. These are predictable, process-oriented and best practice decisions that require little experience or expertise.
- Complicated decisions are those that deal in the known unknowns. The decisions that require analysis and for which there might be a number of different possible outcomes. Complicated decisions are predictable but require expertise to arrive at the answer.
- Complex decisions look at the unknown unknowns. These are decisions where there is cause and effect, but it is not obvious what they are or how they are related – there is a great deal of unpredictability and the outcome can only be deduced in retrospect. A battlefield is a good example of where this applies. There are a near infinite number of decisions that can be made, but in doing so, the situation is immediately changed in unpredictable ways.
- Chaotic decisions are those where cause and effect are unclear and too confusing. The only way to deal with a chaotic decision is to take an action - any action - and try to reduce it to a complex decision.
Understanding these four types of decision helps us to realize where machine learning sits. Because what machine learning can do, better and more consistently than people, is predict. If the answer or outcome to a situation is at all predictable, machine learning can outperform people. Where machine learning is great is with those simple or complicated questions.
Take the example of Google’s AI winning at the Chinese game GO, or IBM’s Deep Blue beating Kasparov at Chess. The AI in these cases was able to look at the games as complicated decisions in which there were knowns (the chess pieces and their moves) and the unknowns (the other player’s move). By analyzing every possible move with more speed and scale than its human opponent, the AI was able to win. Ultimately, the outcomes and decisions AI might make are no different to those of a human. But thanks to unlimited processing power, machine learning can make the decisions faster and more consistently than a human and at greater scale. It can make all of the decisions *now*.
On the flipside, machine learning would not perform well in those decisions that are complex or chaotic, because those are not predictable. These are the kinds of situations that require action and creativity, which is where humans excel.
The financial services industry has managed to grasp this perfectly. Fundamentally, this industry is not that different to the marketing industry – in that there lots of different sources, environmental factors and user behaviours that might impact a course of action. Lots of analysis is required to do the job.
Machine learning has been part of the finance industry for decades. You could say that it started in the 1980s, when Thomas Petterfy changed the stock exchange forever by computerizing trading. Now worth 18 billion dollars, Petterfy’s computerized system was able to make trades far quicker than the standard model - a person and a telephone. It made him the scourge of Wall Street, so much so that his competitors tried to push him out and ban him until they were forced to adopt the same methods.
Today, the financial services industry is still making use of technology, only this time it’s machine learning doing the predictable financial analysis previously done by people.
Companies like Betterment and Money Farm have implemented ‘robo-advisers’, algorithms that instruct clients and manage their portfolios, typically without any additional human interaction. They operate in the simple and complicated space, making decisions based on the analysis of trends and data with predictable outcomes. However, these businesses still have human financial advisors to step in when the decisions become unpredictable. A great example of this is from June 24, 2016, when Betterment suspended algorithmic trading for two and a half hours after the unexpected vote in favour of Brexit sent shockwaves of volatility through the financial markets.
The Robot analyst
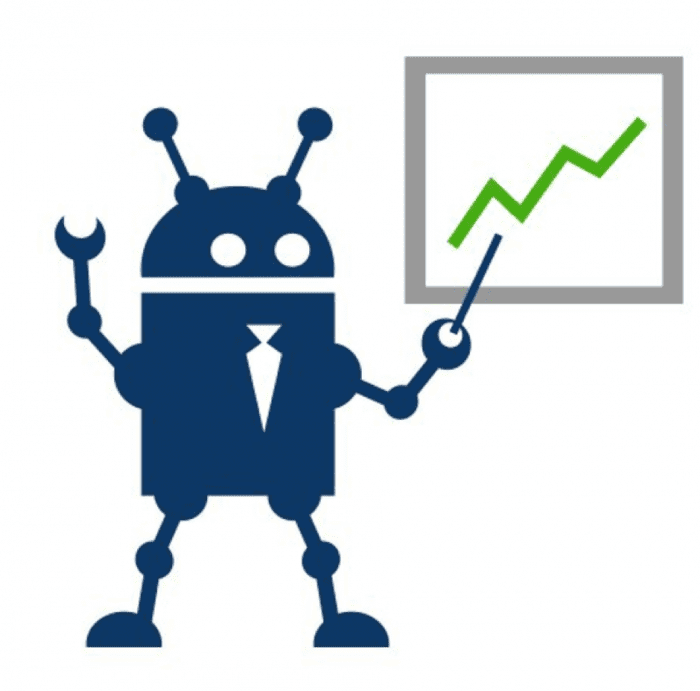
So now we understand what kind of decisions machine learning excels at and how similar industries are applying it to their businesses, let’s look at how it applies to marketing, starting with the original issue. Too much data, in too many places.
Up to now, the way the marketing industry has tried to deal with this issue is by developing more analytics software – but this has only added to the problem. Not only do we have too much data in too many places, we now have too many tools creating even more data in even more places!
What we need and where machine learning can step in, is more analysis NOT more analytics. Think of the financial services example and how it might be applied to your own marketing. In the finance industry, they have robo-advisors, in marketing, why not robo-analysts? There are a large number of repetitive, predictable (and quite likely labour-intensive) tasks that marketers have to perform every week. Data collection, traffic analysis, trend analysis. Tasks that are process-driven and that become more time-consuming every day as more data is created. Tasks that could undoubtedly be actioned by machine learning.
But then there is the unpredictability of marketing, such as Google unexpectedly changing the way search works, a pivot in business direction or a disgruntled customer. These things require human interaction.
In this way, we can fully understand how the combination of expertise and AI can supercharge our marketing. With machine learning and AI to query and report on masses of data, marketers can be kept informed of multiple threats and opportunities, whilst spending time on the creativity and implementation needed to exploit them.
This is something we recognized at Vertical Leap and why we developed Apollo Insights; our machine learning platform that performs all those tasks that can be automated. Apollo audits every page of a website, checking every backlink and checking every query against every page, every week, AND filters data to spot new opportunities for growth. That’s more than is humanly possible, but with robots, algorithms, and AI, we can operate at scale, making sure our focus is not too narrow.
You can also read more about our vision for machine learning analysis in our free download with Smart Insights covering the latest search marketing trends.
This is the future for search marketing
We’ll be hearing a lot about automation, AI and machine learning. There are already discussions about whether search marketing can be completely automated.
Many of the things we do manually may be replaced and done more effectively by robots, but by then we will have evolved further. Search engines will evolve, user behaviour will be different, the way we seek out information will change – marketers will need to adapt with the changes and the only way to keep up will be to look for algorithmic enhancement.
Thanks to Chris Pitt for sharing their advice and opinion in this post. Chris is is head of marketing at
Vertical leap, and has over 20 years’ experience in sales and marketing. He previously held senior roles in tax and financial companies, working with customers such as Ernst & Young, Deloitte and KPMG. He is also a keen musician and plays in a band. You can connect with him on
LinkedIn.